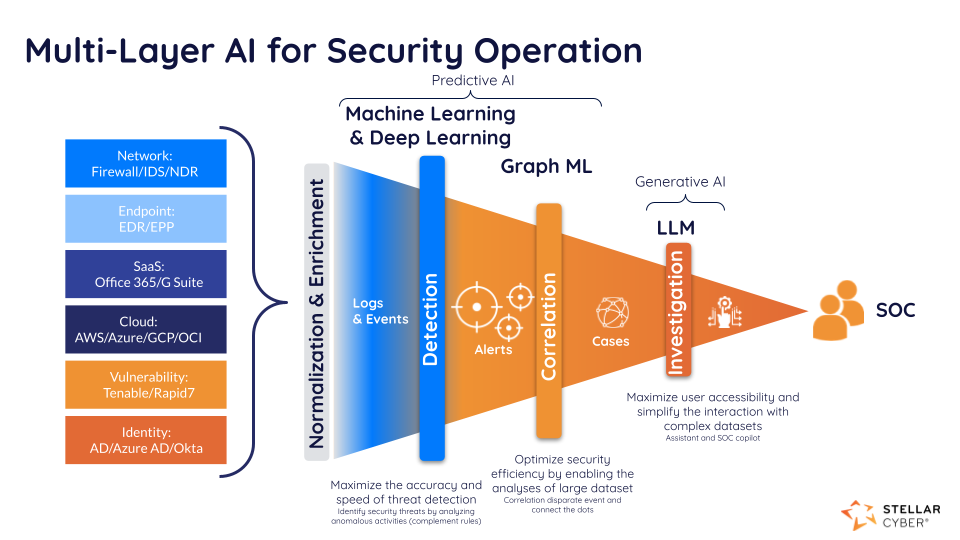
Maximizing Threat Detection Efficacy
Since their founding in 2015, Stellar Cyber has been at the forefront of adopting AI in security operations (SecOps). The company’s mission has always been to make detection and response accessible to all while ensuring that all data, regardless of its source, can be utilized in real-time. This vision has materialized into what is known today as Open Extended Detection and Response (Open XDR). Stellar Cyber’s Open XDR solution ingests security data from any source, ensuring pervasive visibility and enabling robust threat detection. By leveraging unsupervised machine learning, the solution enhances their detection models to identify complex behavioral patterns and anomalies that traditional methods might miss. They also utilize supervised machine learning to detect threats with known patterns such as Domain Generated Algorithms(DGA). These machine learning-based detections are crucial in today’s threat landscape, where sophisticated multi-stage attacks are becoming increasingly common.
Enhancing Operational Efficiency with Correlation, GraphML, and Case-Centric Management
Utilizing Similarity and Correlation
GraphML excels at recognizing similarities and correlations between various entities within your network. By mapping out relationships between data points, GraphML helps detect patterns that might otherwise go unnoticed. For example, it can connect:
- User Entities: Such as Session IDs, Security Identifiers (SIDs), and User Principal Names (UPNs), which can be linked together to detect suspicious user behavior.
- Device Entities: Including device IDs, file names, folders, and registry keys. Correlating activity across devices enables the detection of complex malicious activity spanning multiple endpoints.
- Email Entities: Such as sender and recipient addresses, URLs, and attachments. By correlating email traffic, SOC analysts can detect phishing attempts or email-based attacks.
- Generic Entities: Like IP addresses, cloud resources, and application IDs. Correlating these data points helps uncover coordinated attacks that traverse networks and cloud environments.
Analyzing Causation Through Graph-Based Representations
GraphML also enables causation analysis, which is essential for understanding complex, multi-stage attacks. By analyzing graph-based representations of event data, our system uncovers potential causal relationships between alerts. For example, a phishing email might lead to a compromised endpoint, followed by lateral movement across your network.
This causation analysis allows SOC analysts to trace the progression of an attack and understand the sequence of events, empowering them to respond more effectively. By visualizing the relationships between events, analysts can manage the entire attack flow as a consolidated case rather than dealing with individual alerts in isolation.
Real-World Application:
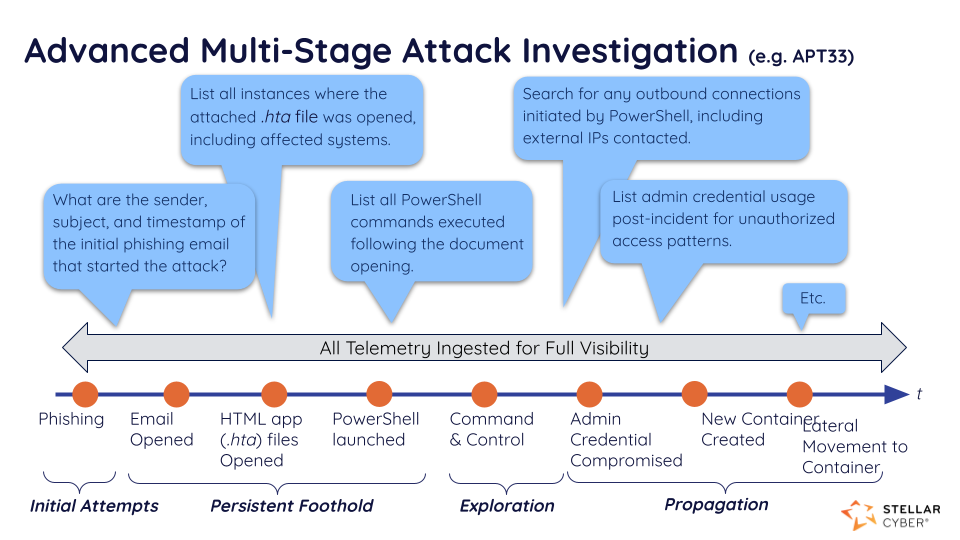
GraphML in Action:
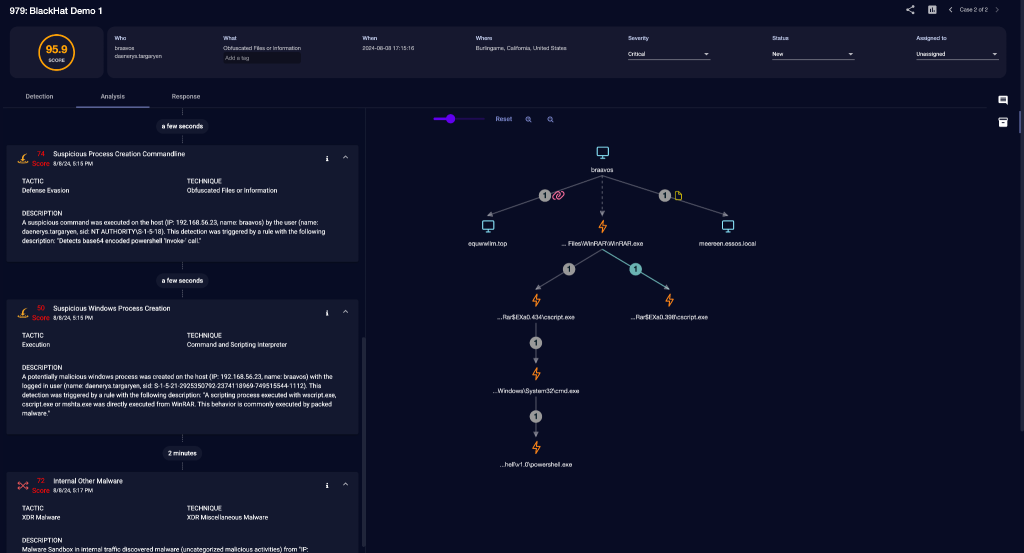
- Automatic Correlation of Alerts: By automatically correlating alerts that share common elements, such as originating from the same host (192.168.56.23) or involving the same entities (bravos, daenerys.targaryen), GraphML simplifies the analysis. This helps in building a cohesive narrative around the attack without manual intervention.
- Holistic View of Attack Vectors: The graph view provided in our XDR platform illustrates the connections between various alerts like suspicious process creation and command line operations leading to the use of PowerShell scripts, which are typical behaviors in sophisticated malware attacks.
- Enhanced Triage Efficiency: With GraphML, SOC analysts are not burdened with isolated alerts but can view an interconnected map of malicious activities, accelerating the triage process. This allows for quicker isolation and remediation of threats, thereby mitigating potential damage.
Operational Benefits Derived:
- Streamlined Detection Workflows: By presenting analysts with a higher-level construct of linked alerts, GraphML aids in faster and more accurate threat detection, cutting down the time required for manual correlation.
- Reduced Alert Fatigue: This technology significantly lowers the number of alerts that need individual attention, reducing alert fatigue and allowing analysts to focus on alerts that truly matter.
- Proactive Threat Hunting: The ability to visualize and correlate attack patterns proactively helps in anticipating potential future attacks based on observed behaviors, enhancing the overall security posture.
Speed Up Threat Investigation with Generative AI
Stellar Cyber is also focused on optimizing the user experience through the integration of Generative AI. Imagine a chatbot that allows security analysts to interact with the system and data using natural language. Similar to ChatGPT but specialized for security investigations, this feature enables analysts to pose questions and describe their tasks naturally.
For example, an analyst might ask, “Identify abnormal behaviors by system administrators outside business hours last week.” The system translates this query into a precise search with all necessary criteria, such as event types, user privileges, and time frames. Analysts can even request visualizations, like “Create a histogram of the top 10 users who received the most phishing attempts,” and the system will generate the chart automatically.
The company’s goal is to ensure that AI integrates seamlessly into human communication methods. By mastering human language, AI can understand nuances and intents, allowing users to focus on their investigations without understanding the complex language of the machine. This natural interaction boosts the efficiency and depth of the investigation process, enabling analysts to create clear mental maps of ongoing situations without worrying about underlying data complexities.
